In this second blog from our deepfake detection series, we explore automated detection tools like TrueMedia.org, Sensity, and Clarity that analyze digital media to distinguish real content from AI-manipulated content. Thus akin to AI-based tools, they may detect inconsistencies in facial movements, lighting, and audio synchronization, providing a starting point for uncovering deepfakes.
However, it’s essential to recognize their limitations and use them with other verification methods such as reverse image search, geolocation, and shadow analysis to ensure robust verification.
While there are plenty of deepfake detection efforts from the industry, there are a couple of projects from academia that have tools open to the public or collaborators like Deepfake-o-Meter from SUNY Buffalo and our own DeFake.app. Both of these tools aggregate deepfake detection models from the research space into a single interface.
Fact-Checking Organizations and Tool Use
Journalistic and fact-checking organizations are increasingly leveraging advanced deepfake detection tools to verify content authenticity before publication. The Deepfake Analysis Unit (DAU), established by the Misinformation Combat Alliance (MCA) in India, has launched a WhatsApp tipline (+91 9999025044) where the public can submit suspicious media for analysis.
This service, available in English, Hindi, Tamil, and Telugu, allows DAU to work with member fact-checking organizations, industry partners, and digital labs to assess and verify content, addressing the growing concern of AI-generated misinformation.
Organizations like WITNESS.org have been focusing on bridging the gap between researchers and practitioners in the field of deepfake detection. They organize meetings between media forensics researchers and fact-checking experts to improve detection methods and work on incorporating emerging detection tools into existing workflows used by journalists and open-source investigators.
WITNESS emphasizes the need for detection approaches that are accessible and understandable to real-world users in journalism and human rights, prioritizing input from diverse global users.
Northwestern University’s Global Online Deepfake Detection System (GODDS) provides a free platform for journalists to submit digital artifacts for analysis, combining automated algorithms with human expertise to render opinions on potential deepfakes within 24 hours. This system has been particularly valuable for smaller news organizations that may lack extensive in-house technical resources.
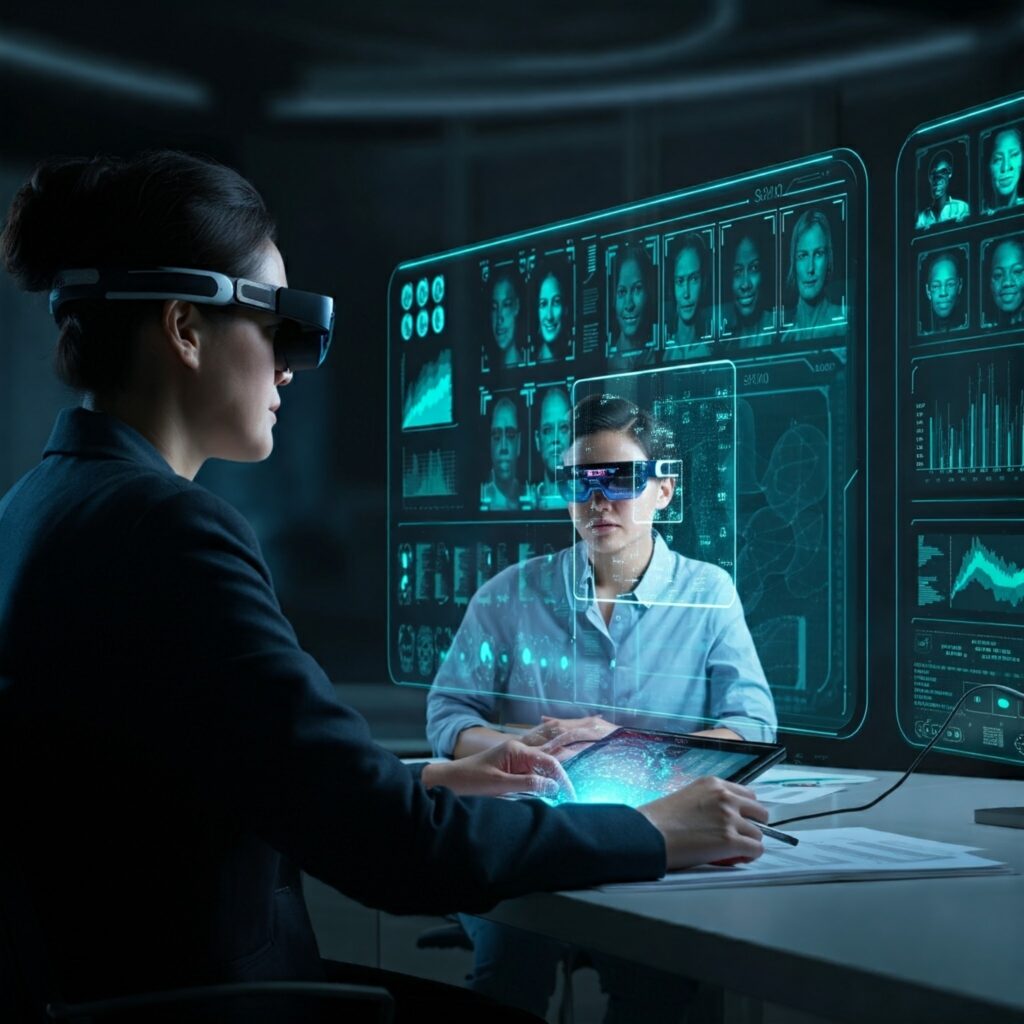
Meanwhile, larger media conglomerates like Agence France-Presse (AFP) have integrated AI-powered tools into their fact-checking units, allowing them to quickly analyze potentially manipulated content across their global network.
Similarly our own, DeFake Project, is an academic open-source initiative that provides a free deepfake detection tool accessible via a user-friendly web interface. This project focuses on providing access to not only journalists and fact-checkers but also forensic analysts and law enforcement.
The DeFake project utilizes advanced machine learning algorithms to analyze images and videos, providing a probability score for whether the content is authentic or manipulated as well as providing expertise to news organizations like previously mentioned DAU.
Organizations like Bellingcat have been at the forefront of using and promoting advanced digital forensic techniques for content verification. Bellingcat employs various tools including metadata analysis, reverse image searches, and satellite imagery analysis, often collaborating with other organizations to improve their detection methods.
Recently, Bellingcat launched its Online Investigations Toolkit, a collaborative platform open to contributions from the wider open-source researcher community. This toolkit aims to help investigators find and learn how to use various investigative tools across categories such as satellite imagery, maps, social media, transportation, and archiving.
Meanwhile, the role previously filled by First Draft in training journalists has been taken up by other organizations. For instance, the Information Futures Lab at Brown University has incorporated some of First Draft’s initiatives, continuing the work of educating journalists on digital verification techniques.
Additionally, organizations like the Poynter Institute and its International Fact-Checking Network (IFCN) have stepped up to offer training and resources for journalists and fact-checkers in detecting manipulated media. These efforts collectively contribute to a growing ecosystem of tools and knowledge aimed at combating misinformation and verifying digital content in an increasingly complex media landscape.
Reality Defender and WeVerify have developed platforms specifically designed for media organizations and fact-checkers, employing multi-model approaches to detect various types of manipulated content. News agencies and fact-checking organizations have integrated these platforms into their workflows, allowing for scalable detection that can handle large volumes of content. This integration has been particularly crucial for media organizations dealing with the rapid spread of information on social media platforms.
The trend towards broader accessibility of deepfake detection tools is evident in various initiatives across the industry. TrueMedia.org, for instance, aims to integrate its detection capabilities directly into web browsers and social media platforms, potentially allowing users to verify content in real-time as they browse.
Sensity offers integration with browser extensions and OSINT tools, primarily targeting intelligence and security applications. DuckDuckGoose AI focuses on providing detection software that can be integrated into existing systems for content moderation and verification.
The DeFake Project aligns with this accessibility trend by offering a web-based tool that can be easily accessed and used without specialized technical knowledge, extending access to deepfake detection technology for our collaborating users. These diverse approaches reflect the growing recognition of the need for user-friendly, widely available tools to combat the spread of manipulated media across various digital platforms.
Tool Limitations and Accuracy Concerns
AI-based deepfake detection tools often rely heavily on the quality and type of input data. Data quality can greatly affect results. Poor quality inputs can lead to inconsistent or inaccurate detection. For instance, a tool might provide opposite results if the resolutions of the video is low. Additionally, heavy post-processing used to enhance or compress media can destroy telltale artifacts, potentially leading to misclassification.
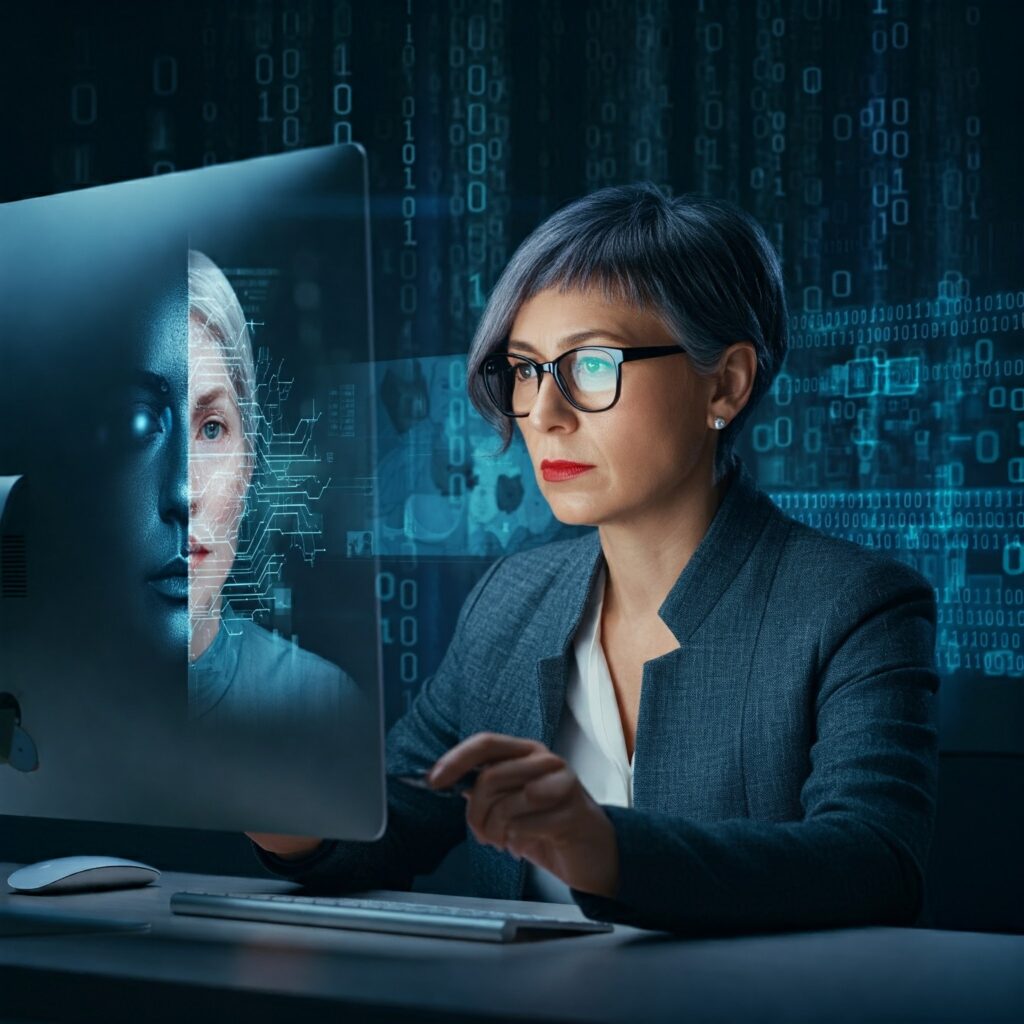
Detectors also struggle with the gap between training data and “in-the-wild” deepfakes, which can limit the tool’s effectiveness in practical scenarios. Training datasets often consist of examples curated to capture known deepfake patterns, but real-world deepfakes evolve rapidly and may present features that these models have never encountered.
Moreover, recent research suggests that the research datasets do not appropriately represent the real world distribution of fake vs real videos, which may lead to these models predicting a lot more real videos as fakes. These challenges are compounded by adversarial techniques, where intentional noise is added to fool detectors and cause misclassification.
Ethical considerations also come into play when deploying AI-based deepfake detectors. These tools may inadvertently flag legitimate content or exhibit biases, raising concerns about false positives and potential misuse. There are also privacy implications to consider when processing user-submitted media through detection systems. Ethical deployment requires balancing the benefits of detection with transparency, accountability, and careful consideration of the societal impacts.
To address these limitations, ongoing research focuses on improving detector robustness, expanding training datasets, and developing multimodal approaches that analyze visual, audio, and text cues. However, the cat-and-mouse game between deepfake creators and detectors is likely to continue, necessitating a combination of technological and human-centered approaches to combat synthetic media threats.
Free vs Paid Tools
Understanding the range of available tools, both free and paid, is crucial for journalists and fact-checkers. Each type of tool offers different capabilities and is suited to different needs and resources. Here’s a breakdown of commonly used tools:
Free Tools:
- TrueMedia: Can be used by anybody and everybody to be able to detect deepfakes in real-time
- ElevenLabs’ Speech Classifier: Analyzes AI-generated audio; useful for detecting voice cloning in public figures.
- AI or Not: Helps identify whether an image was generated by AI, offering a simple drag-and-drop interface.
- Google Reverse Image Search: Cross-references images to verify authenticity.
- GODDS: A Northwestern Security & AI Lab effort to provide deepfake detection resources to journalists.
- DeepFake-O-Meter: A University of Buffalo effort to provide deepfake image, video, and audio detection resources to the public.
- DeFake.app: A ESL Global Cybersecurity Center effort to provide deepfake detection and digital media forensics resources to journalists, forensic analysts, and law enforcement.
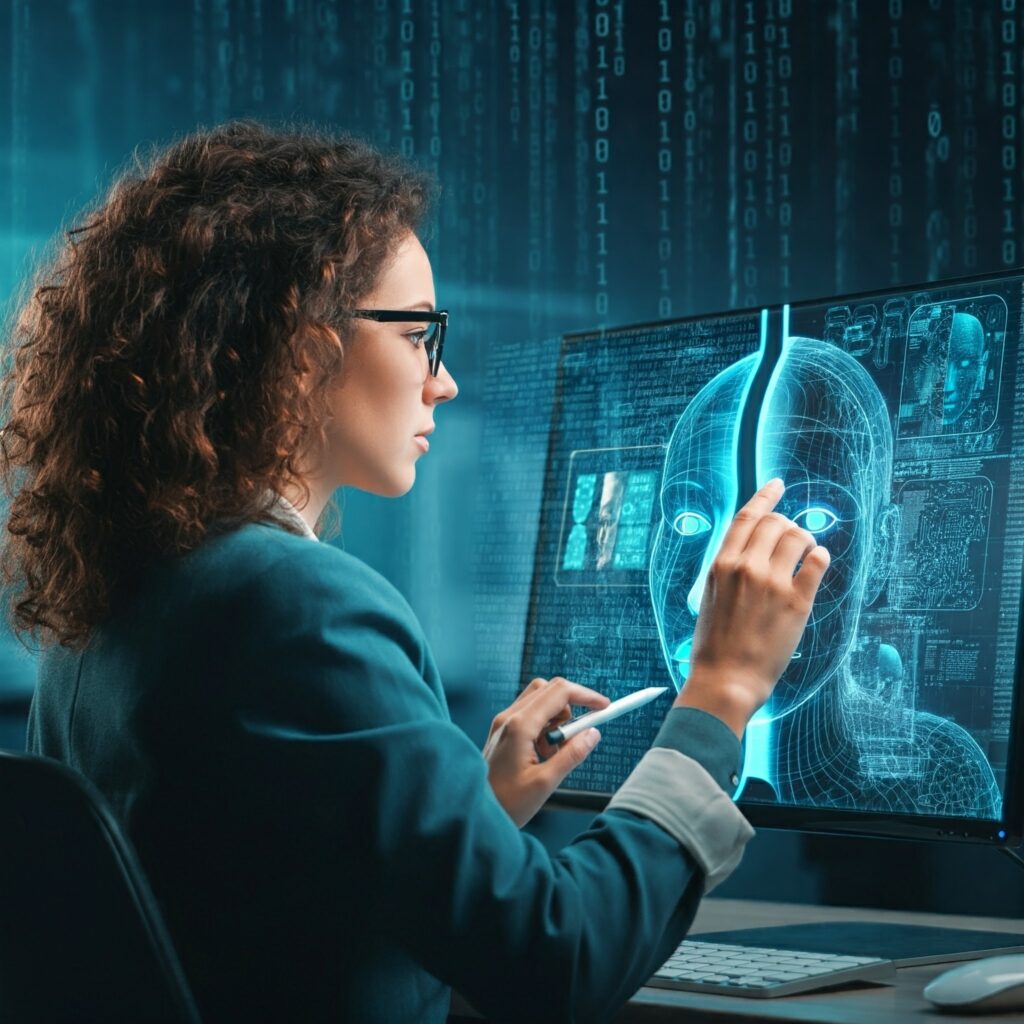
Paid Tools:
- Sensity: Specializes in detecting face swaps and manipulated audio.
- Hive Moderation: Offers detection for both images and videos, with advanced AI models tailored for security purposes.
- Reality Defender: An enterprise-level deepfake detection platform for governments and major media houses.
As deepfake technology continues to evolve, so too must our detection methods. The tools and organizations discussed in this post represent the current state of deepfake detection, but ongoing research and collaboration between academia, industry, and fact-checking organizations will be crucial to stay ahead of increasingly sophisticated manipulation techniques.
While AI-powered tools provide valuable assistance, they are most effective when combined with human expertise and traditional verification methods. The future of deepfake detection lies in this synergy between technological innovation and journalistic diligence.